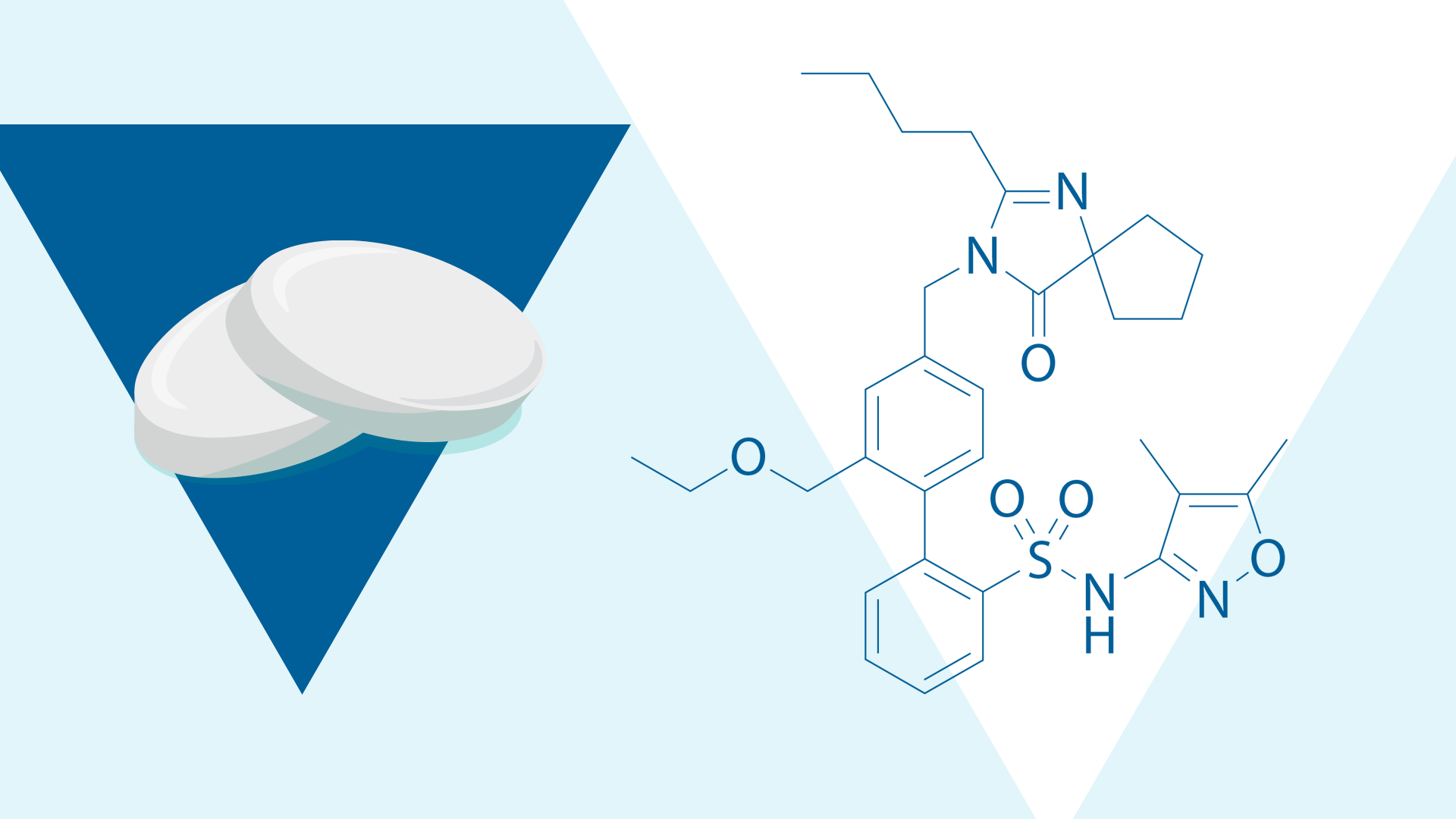
Physiologically-Based Pharmacokinetic Model of Sparsentan to Evaluate Drug-Drug Interaction Potential
Topics: Nephrology FSGS IgAN DDI Sparsentan
Patel NK, Chen K-F, Chen S-C et al.
Clinical Pharmacology and Therapeutics Pharmacometrics & Systems Pharmacology
View Publication
10.1002/psp4.13086
Summary
A bottom-up physiologically-based pharmacokinetic (PBPK) model can predict pharmacokinetics (PK) and drug-drug interactions (DDIs) of sparsentan1
Background
Physiologically-based pharmacokinetic (PBPK) models can be used to predict pharmacokinetics (PK) and drug-drug interactions (DDIs) of based on physiological, chemical, preclinical, and clinical data.2
Sparsentan is a dual endothelin type A receptor (ETAR) and angiotensin II subtype 1 receptor (AT1R) antagonist3; in vitro studies of sparsentan show that it may induce or inhibit the activity of cytochrome P450 (CYP) enzymes, which play a key role in the metabolism and clearance of many drugs.1,4 In particular, sparsentan is thought to inhibit and induce CYP2C9, CYP2C19, and CYP3A4.1
Aim
The aim of this study was to develop and verify a PBPK model of sparsentan PK and simulate the potential DDIs for sparsentan as both a victim and perpetrator.1
Approach
Model development
A PBPK model was developed using Simcyp™ PBPK Simulator (a software package for predicting drug behavior in the human body).1 This model incorporated in vitro data including1:
- Solubility and permeability
- Formulation dissolution and particle size
- Metabolic pathways
- Inhibition/induction of metabolic enzymes
- P-glycoprotein (P-gp) driven efflux
Model verification and application
The PBPK model was verified using data from various clinical studies of sparsentan, specifically1:
- Four clinical studies
- A single and multiple ascending dose study in healthy volunteers
- A study of food and fasting effects in healthy volunteers
- A study of the effect of itraconazole on sparsentan PK (to verify the effect of CYP3A4 metabolism on sparsentan)
- A study of the effect of sparsentan on the PK of midazolam in healthy volunteers
The verified model was used to simulate DDIs of sparsentan with several drugs not studied in the clinical trials.1
Findings
Under various conditions, the validated PBPK model predicted PK and DDIs of sparsentan that were consistent with data from clinical studies1
The model predicted plasma concentration-time profiles for sparsentan consistent with data from clinical studies in single and multiple doses (ranging from 50-1600 mg) and could also predict food effects.1
Sparsentan is a victim and perpetrator of CYP3A4 DDIs1
The model also consistently predicted the observed effect of itraconazole on sparsentan (increased exposure) and the minimal interaction of sparsentan and midazolam reported in clinical studies.1
Sparsentan can act as DDI victim or perpetrator1
DDI modeling of sparsentan as a victim showed sparsentan exposure within two-fold of control for all inducers/inhibitors at both steady-state or a single dose, except for fluconazole.1
Predicted area under the curve (AUC) DDI-to-control ratio:
- Steady-state sparsentan + fluconazole: 2.23
- Single dose (800 mg) sparsentan + fluconazole: 2.33
As a perpetrator, sparsentan 800 mg at steady state had no or minimal impact on predicted exposures on the CYP3A4 and CYP2C19 substrates, midazolam or omeprazole.1
P-gp inhibition had a negligible effect on sparsentan1
Simulations of complete inhibition of P-gp-mediated transport in the gut and liver showed a negligible effect on sparsentan exposure.1
Key takeaway
This PBPK model evaluated the interactions between CYP450 enzymes and sparsentan as either a perpetrator or victim and contributed to the growing knowledge of DDIs.1 This model may be helpful in evaluating the risks of DDIs of sparsentan in people with IgA nephropathy.1
Footnotes
AT1R, angiotensin II subtype 1 receptor; AUC, area under the curve; CYP, cytochrome P450; DDI, drug-drug interaction; ETAR, endothelin type A receptor; IgA, immunoglobulin A; PBPK, physiologically-based pharmacokinetic; P-gp, P-glycoprotein; PK, pharmacokinetics.
- Patel NK et al. CPT Pharmacometrics Syst Pharmacol. 2024;13(2):317-329.
- Min JS, Bae SK. Arch Pharm Res. 2017;40(12):1356-1379.
- Kowala MC et al. J Pharmacol Exp Ther. 2004;309(1):275-278.
- Pan-Zhou XR et al. J Am Soc Nephrol. 2016;27(suppl):746A (Abstract).
MA-SP-24-0098 | September 2024